Projekte
Laufende Projekte
Modeling – Analysis – Optimization of discrete, continuous, and stochastic systems
Projektleitung
Projektmitarbeiter:innen
Laufzeit
01.10.2020 - 30.09.2025
Förderung
Fonds zur Förderung der wissenschaftlichen Forschung (FWF)
Webseite
Optimization problems accompany us all the time in our every-day life. For example supermarkets guarantee the supply by optimizing the route of transportation of their goods, electricity providers optimize the supply with electricity, and highways are built in a way such that cars produce as little noise as possible. For this, discrete, stochastic, which means influenced by randomness, and continuous mathematical models are used, which need to be analyzed. In order to solve such problems, it is often essential to have a multi-perspective view and combine the knowledge of several mathematical sub-disciplines to create synergies. In crossing these borders lies a great innovative potential. It is the aim of the doc.funds doctoral school and its nine professors from the Departments of Mathematics and Statistics at the University of Klagenfurt to provide PhD students with the mathematical knowledge necessary for understanding and solving challenging mathematical questions, coming from optimization problems in every-day life.
High-dimensional statistical learning: New methods to advance economic and sustainability policies
Projektleitung
Gregor Kastner, Laura Vana, Florian Huber, Philipp Piribauer, Laura Nenzi, Karin Dobernig, Stefan Schupp
Projektmitarbeiter:innen
Luis Bastian Gruber, Alexander Mozdzen, Florian Schwendinger, Annalisa Cadonna, Laura Vana, Rainer Hirk, Florian Huber, Michael Pfarrhofer, Niko Hauzenberger, Philipp Piribauer, Laura Nenzi, Roman Kuznets, Ennio Visconti, Karin Dobernig, Stephan Adelsberger, Roman Parzer, Camilla Damian, Nico Amstätter-Zöchbauer, Stephan Adelsberger
Laufzeit
01.08.2019 - 31.07.2024
Förderung
Fonds zur Förderung der wissenschaftlichen Forschung (FWF)
Webseite
Recent years have seen a tremendous surge in the availability of socioeconomic data characterized by vast complexity and high dimensionality. However, prevalent methods employed to inform practitioners and policy makers are still focused on small to medium-scale datasets. Consequently, crucial transmission channels are easily overlooked and the corresponding inference often suffers from omitted variable bias. This calls for novel methods which enable researchers to fully exploit the ever increasing amount of data. In this project, we aim to investigate how the largely separate research streams of Bayesian econometrics, statistical model checking, and machine learning can be combined and integrated to create innovative and powerful tools for the analysis of big data in economics and other social sciences. Thereby, we pay special attention to properly incorporating relevant sources of uncertainty. Albeit crucial for thorough empirical analyses, this aspect is often overlooked in traditional machine learning techniques which have mainly been centered on producing point forecasts for key quantities of interest only. In contrast, Bayesian statistics and econometrics are based on designing algorithms to carry out exact posterior inference which in turn allows for density forecasts. Our contributions are twofold: From a methodological perspective, we develop cutting-edge methods that enable fully probabilistic inference of dynamic models in vast dimensions. In terms of empirical advances, we apply these methods to highly complex datasets that comprise situations where either the number of observations, the number of potential time series and/or the number of variables included is large. More specifically, empirical applications center on four topical issues in the realm of sustainable development and socioeconomic policy to answer questions such as: How do market and economic uncertainty affect income inequality? What are the relationships between greenhouse gas emissions and macroeconomic indicators? Which role do tweets play in the evolution of the prices of crypto-currencies? Which policy measures are most effective to foster sustainable urban mobility patterns? In these applications, we focus on probabilistic forecasting using real-time data to perform model validation in an efficient way. Moreover, we address structural inference. As policy makers are typically interested in evaluating their policies quantitatively, robust econometric tools are crucial for counterfactual simulations. In light of the increasing complexity of the economy, however, large information sets need to be exploited to appropriately recover the underlying causal structures and provide a rich picture of potential transmission channels of policy interventions. The team constitutes a genuinely collaborative partnership of five young high-potential researchers composed of statisticians, machine learning experts, macro- and regional economists as well as social and computer scientists.
Eine vollständige Liste aller Forschungsprojekte des Instituts für Statistik finden Sie in der Forschungsdokumentation (FoDok).
Abgeschlossene Projekte
Integrated Development 4.0
Projektleitung
Projektmitarbeiter:innen
Laufzeit
01.05.2018 - 31.12.2022
Förderung
Horizon 2020, Österreichische Forschungsförderungsgesellschaft mbH (FFG)
Task 1.2.1 – Capture the Competences and information Flow: Development of intelligent statistical data pre-processing methods for semiconductor manufacturing. Probabilistic graphical modeling, in close cooperation with the group of Prof. Reiner, will be used to infer the dependence structures and dimension reduction schemes. Task 1.2.2 – Dynamic Knowledge Update: Development of intelligent learning algorithms to extract relevant information out of big data sets with a focus on adaptive and networked (smart) production systems, in cooperation with the group of Prof. Reiner. Particular attention will be paid to Bayesian regularization methods, Extract key parameters for process control from results of Statistical Machine Learning and Bayes Deep Learning algorithms, in close cooperation with the KnowCenter group, Development of Bayesian ensemble filtering and data assimilation methods incorporating the observations and process dynamics through sequential posterior updating (modified Bayes Kalman filters), Monitoring of probability distributions as data summaries instead of only using selected key numbers of raw dat, Combination of methods of active learning and model choice to take account of covariate shift (Bayes statistical learning in non-stationary environments). Task 1.2.3 – Knowledge Validation: Validation of KPIs for decision making support with a focus on adaptive and networked (smart) production systems, in close cooperation with the group of Prof. Reiner:Validation of key parameters to increase acceptance of data driven methods in semiconductor manufacturing environment, Validation of knowledge about dependencies between advanced dynamic screening methods and production system performance: In particular, we will investigate the use of empirical Bayes estimation of posterior probabilities of enrichment for controlling the False Discovery Rate (FDR). Task 1.3.2 – Data Driven Methods (AI, Deep Learning, Black-Box Modeling, etc.): Development and integration of data driven methods to support and enable an effective root cause analysis of yield loss (Functional ANOVA Decompositions, Approximate Inference Algorithms), Development of novel Bayesian variational and perturbation methods and their integration into structured predictors and deep learners of production performance characteristics (Bayes deep learning). Task 1.3.3 - Validation of AI approaches: Jointly with KAI and the group of Prof. Reiner, we will work on the validation of the implemented routines from Task 1.3.2 by comparing expert results and results of data driven methods with regard to accuracy, robustness, etc. With regard to the joint work on the tasks within UC1 (WP 1, 4), we will further focus on relating (raw data) machine parameters and SPC parameters through Canonical Correlation Analysis and the choice of a “best” set of training data.
Planung und Instandhaltung eines Sensormessnetzes für Umweltdaten für das Görtschitztal
Projektleitung
Projektmitarbeiter:innen
Albrecht Gebhardt, Maximilian Arbeiter
Laufzeit
01.12.2016 - 31.12.2020
Im Görtschitztal soll ein privates Sensormessnetz für Umweltdaten wie Luftfeuchtigkeit, Temperatur, Druck, Wind, Niederschlag, Nebel, Lichtintensität, NO2, S02, CO, CO2, O2, NHO§, NH3, PM und Schwermetalle aufgebaut und instand gehalten werden. Die elektronische Sensorik basiert auf Arduino, Waspmote und Raspberry. Zum Nachvollziehen der Umweltbelastungen kommen Methoden der Räumlichen Statistik und Umweltstatistik zum Einsatz.
Numerical methods for stochastic differential equations with irregular coefficients with applications in risk theory and mathematical finance
Vergabe einer Diplomarbeit mit dem Arbeitstitel „Insurance Education“
Projektleitung
Laufzeit
01.09.2019 - 31.03.2020
Förderung
Kärntner Gesellschaft für Versicherungsfachwissen (KGV)
Erstellung einer Diplomarbeit zum Thema Insurance Education in Sekundarstufe I, Sekundarstufe II und in der Erwachsenenbildung. Wie funktioniert Versichern und auf welchen mathematischen Grundlagen basiert es? Diese Fragen sollen für die unterschiedlichen Altersstufen geklärt werden und es sollen Unterrichtsmaterialien entwickelt werden.
Hydrometeorological and particle dispersion data at the Worthersee (Klagenfurt)
Projektleitung
Projektmitarbeiter:innen
Laufzeit
01.01.2018 - 31.03.2019
Researchers from Geography and Statistics at the Alpen-Adria-University aim to promote crowd meteorological data collection in Klagenfurt am Wörthersee to investigate local spatial differences and particle distribution across the city in function of the meteorological conditions using mathematical models. An initial amount of 10 meteorological stations will be installed by staff and students from the University starting in 2018 onwards. Through user-friendly weather stations and the Internet of Things technology, “amateur” users can download automated sub-hourly observations, store electronically and carry on easy analysis and data sharing through an online platform.Members of the project will carried out local analytics and after aggregation of the information applications to smart cities, smart environment, security, smart metering and smart agriculture would be possible.
EPT300
Projektleitung
Projektmitarbeiter:innen
Laufzeit
01.04.2012 - 08.11.2016
Förderung
ENIAC JU, Österreichische Forschungsförderungsgesellschaft mbH (FFG), Infineon Technologies Austria AG
Entwickelt werden statistische Methoden und Verfahren zur Prozesskontrolle für die neue 300mm Wafer-Technologie zur Herstellung von Chips für die Automobil- und Industrieelektronik.
Kooperationspartner:innen
Snapshot Spectral Imaging
Projektleitung
Projektmitarbeiter:innen
Laufzeit
01.02.2009 - 31.03.2013
Förderung
FFG - Basis-Programm
Im Gegensatz zu den klassischen sequentiellen SI-Aufnahmeverfahren ermöglichen Snapshot Spectral Imaging-Verfahren die Erfassung der räumlichen und der spektralen Informationen durch die Aufnahme eines einzelnen Bildes. Ziel des Projekts ist die Entwicklung statistischer Methoden und Algorithmen zur Trennung überlappender Emissionsspektren (spectral unmixing), insbesondere für Vielkanalanwendungen. Als diagnostische Anwendung ist vom Projektpartner Tissue Gnostics (Wien) der Bereich der Prostatakrebserkennung vorgesehen; die Projektkoordination erfolgt duch das CTR Villach.
Quicklinks
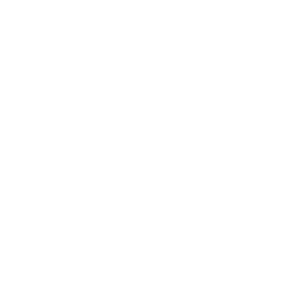
Informationen für
Adresse
Universitätsstraße 65-67
9020 Klagenfurt am Wörthersee
Austria
+43 463 2700
uni [at] aau [dot] at
www.aau.at
Campus Plan