
Learning from and for Heterogeneous and Ambiguous Data
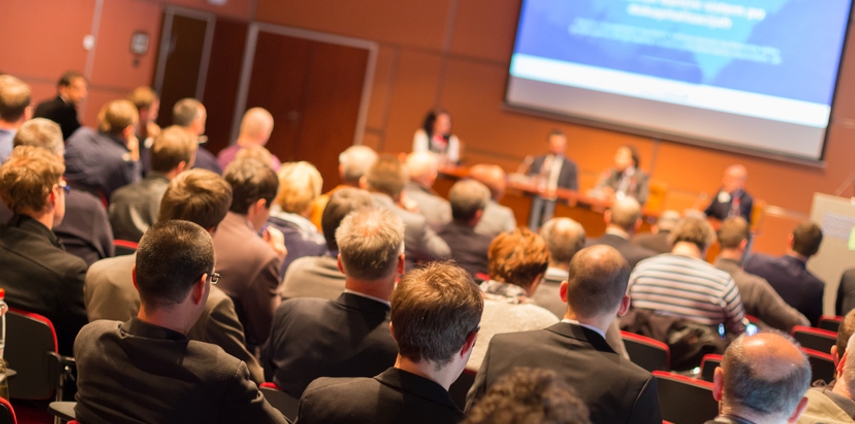
When talking about new developments in Machine Learning, we typically think about new algorithms, better optimization techniques, or optimized hyperparameters. However, one important aspect is often neglected: the quality and the structure of training data: measurement noise, label noise, and correct but ambiguous labels. In this talk, we address the latter problem, trying to deal with high intra-class and small inter-class variability in the data, following two different strategies. First, we consider the problem of metric learning, showing that by selecting/learning a better metric for a specific problem, better results can be obtained: using the same learning method and the same data. Second, focusing on neural networks, we analyze the influence of specific hyperparameters, namely the activation functions. For both directions, we show that the quality of the finally learned model is highly dependent on the data. To illustrate these aspects, we will further discuss a visualization technique, namely information planes, providing better insights into the current state of the learning system.
Veranstalter
Vortragende(r)
Univ.-Prof. DI Dr. Peter M. Roth
Kontakt
Christian Timmerer (christian [dot] timmerer [at] aau [dot] at)