
Vortrag im Rahmen des Doctoral Seminar in Mathematics von Herrn Timo Welti (University of Vienna)
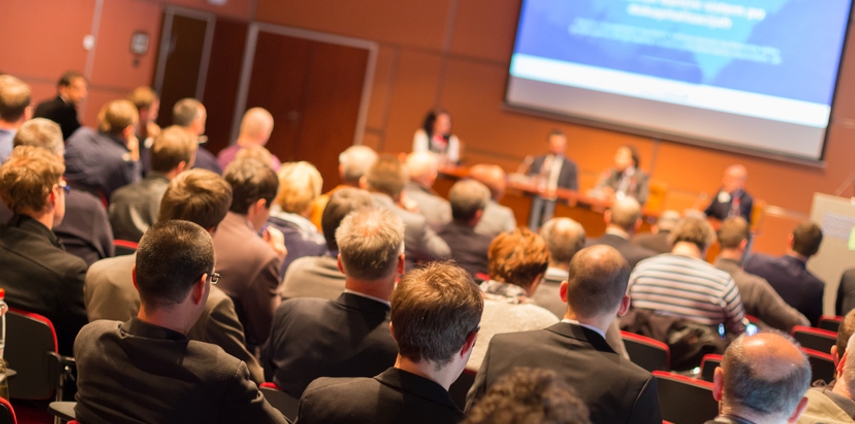
Veranstaltungsort
I.2.01
Veranstalter
Institut für Mathematik
Beschreibung
Titel:
Why deep artificial neural networks overcome the curse
of dimensionality in PDE approximation
Kurzfassung:
In recent years deep artificial neural networks (DNNs) have very successfully
been employed in numerical simulations for a multitude of computational
problems including, for example, object and face recognition, natural
language processing, fraud detection, computational advertisement, and
numerical approximations of partial differential equations (PDEs). Such
numerical simulations indicate that DNNs seem to be able to overcome the
curse of dimensionality in the sense that the number of real parameters used
to describe the DNN grows at most polynomially in both the reciprocal of the
prescribed approximation accuracy and the dimension of the function which
the DNN aims to approximate in such computational problems. While there
is a large number of rigorous mathematical approximation results for artificial
neural networks in the scientific literature, there are only a few special
situations where results in the literature can rigorously explain the success
of DNNs when approximating high-dimensional functions. In this talk it is
revealed that DNNs do indeed overcome the curse of dimensionality in the
numerical approximation of Kolmogorov PDEs with constant diffusion and
nonlinear drift coefficients. The presented ideas for proving this crucially
Vortragende(r)
Timo Welti
Kontakt
simone gahleitner (simone [dot] gahleitner [at] aau [dot] at)